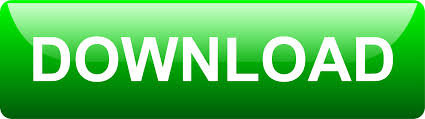
This system makes the signal detection problem a big challenge, because the computational complexity of the detector increases with the number of antennas. Massive multi-input multi-output (MIMO) is a key technology in B5G, where tens, hundreds or thousands of antennas are equipped at the base station. With the rapid development of machine learning techniques, especially deep learning techniques, it is crucial to consider symbol error ratio (SER) and complexity when applying algorithms. These algorithms are also being used in communication technologies to improve system performance in terms of spectrum utilization, latency and security. In recent years, machine learning algorithms have been used in various fields such as healthcare, transportation, energy, and self-driving cars. Artificial intelligence technology is the engine of B5G. The simulation results show that, compared with the existing model-driven algorithms, BGS-Net has lower complexity and similar the detection performance good robustness, and its performance is less affected by changes in the number of antennas Improved BGS-Net can improve the detection performance of BGS-Net.īeyond fifth generation (B5G) is a perfect process to solve some application scenarios and technologies of 5th generation mobile communication technology (5G). In order to improve the symbol error ratio (SER) of BGS-Net under MAUE system, we propose Improved BGS-Net. We reduce complexity by converting a large matrix inversion to small matrix inversions. In order to reduce the high complexity of parallel running of the traditional Gauss-Seidel iterative method, this paper proposes a model-driven deep learning detector network, namely Block Gauss-Seidel Network (BGS-Net), which is based on the Gauss-Seidel iterative method. In massive multiple-input multiple-output (MIMO) systems with single- antenna user equipment (SAUE) or multiple-antenna user equipment (MAUE), with the increase of the number of received antennas at base station, the complexity of traditional detectors is also increasing.
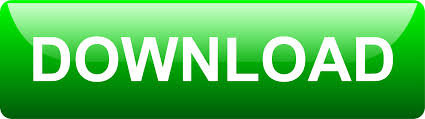